Crawl, walk, run: An inside look at Clearbit's lead qualification evolution
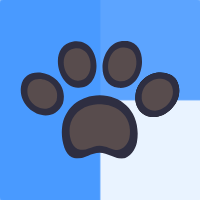
Since Clearbit was founded in 2015, our approach to lead qualification has evolved along with our scale, resources, and goals.
What began as just a couple salespeople manually picking through leads has expanded into a dynamic force comprised of 50+ sales and 25+ customer success teammates in a 150+ person company. And we need to supply them all with great leads.
Today, Clearbit has multiple products, customer types, and go-to-market (GTM) strategies, so it only makes sense that the way we score and route leads for our sales team would change, too.
We can see these changes over time reflected in four areas of lead qualification:
- Team size and structure
- Type of lead score
- Tools and tech stack
- ICP, or ideal customer profile
We’ll explore these one by one, starting with our team, which grew and gained complexity as our company’s needs changed.
1. Team size and structure
A few years ago, our sales team was in the crawl phase, as John Delich, our head of RevOps describes it. We had one core group of SDRs working as many leads as possible, eliminating obvious no’s. As is the case for most SaaS startups, the early days were all about hustle. It was an important time for figuring out who our customers really were, what we were really selling, and how best we could go about selling it.
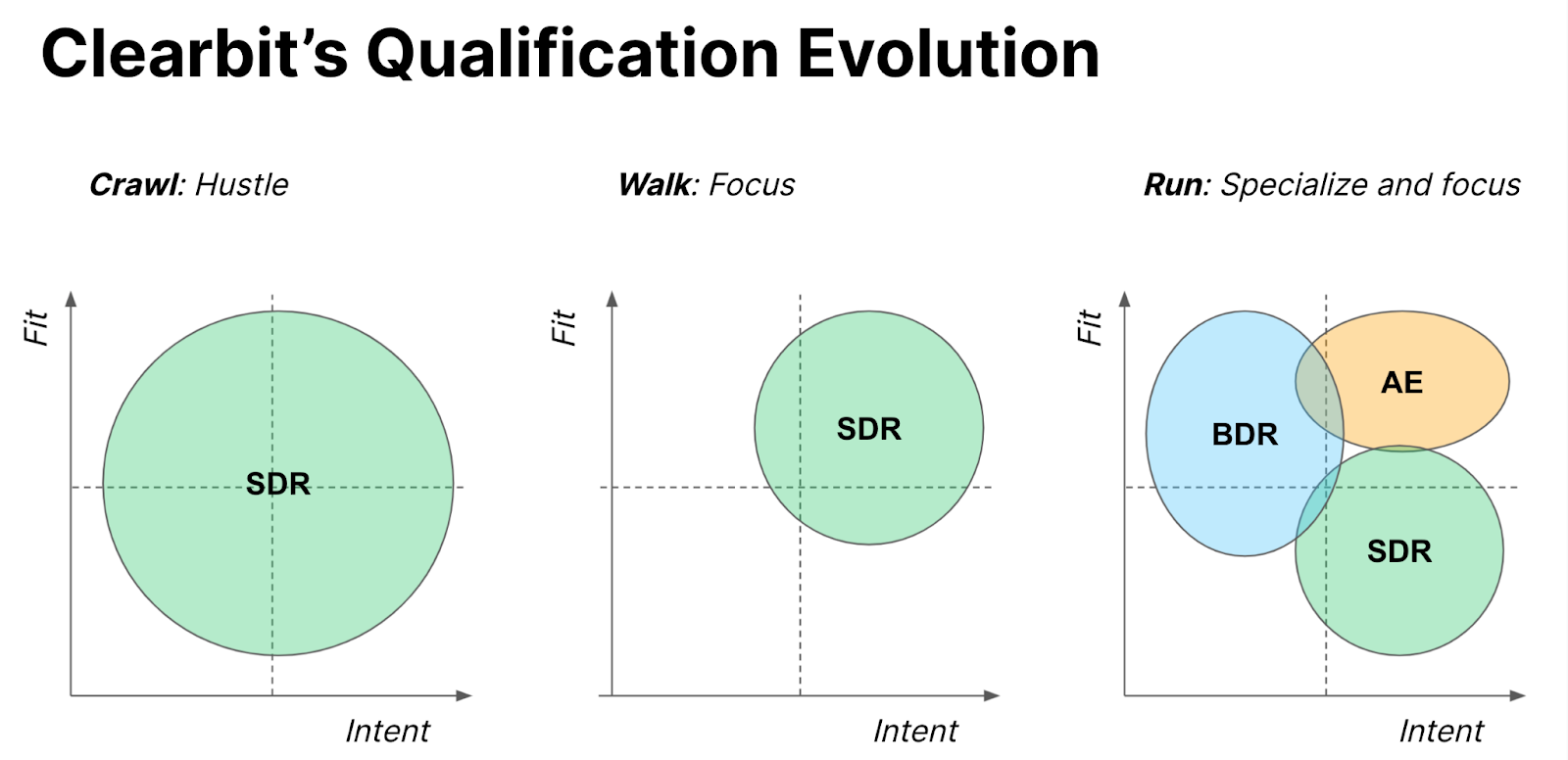
The next phase, walk, was all about focusing our SDR team’s attention: They devoted their time to pursuing leads who were both high fit and high intent. Behind the scenes, marketing worked on the rest of the leads, warming up the good-fit ones until they were ready to talk to sales.
The final phase, and where we are today, is run. It’s about both focus and specialization, so we have multiple sales teams that focus on different things. AEs work the high-intent, high-fit leads. BDRs proactively reach out to educate and pull in high-fit leads that aren’t yet initiating conversations with Clearbit. Meanwhile, the SDR team is responsible for vetting low-fit leads that show high intent — for example, a company that’s requested a demo but falls outside Clearbit’s typical industry targets. SDRs qualify these leads before involving the AEs, who are our most specialized and expensive resource.
This evolution has given us room to refine our approach to the different types of leads in our market, allowing us to deploy our sales resources in the most cost-effective way.
2. Type of lead score
Today, our lead qualification is based on predictive scoring powered by machine learning. But we landed here by navigating through a series of lead score types — from a simple yes/no score to a point-based score to a predictive one. We’ve also expanded scoring to incorporate more and more data points over time.
At first, sales reps manually filtered out leads that were obviously spam or attributable to individuals (as identified by Gmail addresses not linked to business accounts), making “scoring” a rather binary affair. Over time, they identified a few firmographic attributes, such as company size and industry, to help prioritize those leads.
At just about three years old, our prioritization got more nuanced. Armed with a shortlist of attributes that our closed won customers had shared, Clearbit was able to create a formal point-based score that dropped each lead into an A, B, or F-score bucket.
Higher scores were routed to AEs, while SDRs vetted the lower scores. Built in Salesforce Process Builder, the scoring model’s logic assigned the A, B, or F score to the lead’s record in Salesforce, where reps could easily see it and sort their lists by priority.
To achieve a point-based score, we first identified the data points that would feed the score: Would it be company size, industry, both, something else? To find out, we ran a regression analysis on our closed-won customers, resulting in a CSV file that highlighted the attributes shared by more than 50% of all customers. It was a simplistic formula, but it still revealed great insights that helped us focus our energy and eventually formed the basis for our ICP.
The formula landed on a handful of Clearbit fit points for the point-based score, including:
- Firmographic:
- Industry
- Business model tags (e.g., SaaS, B2B, etc.)
- Technology used (e.g., Salesforce, Marketo, Drift, etc.)
- Estimated annual revenue
- Employee range
- Country
- Demographic:
- Sales/marketing team
- Leadership level (indicator of purchasing power)
Our lead scoring assigned points to these different attributes. For example, a US-based B2B SaaS finance company that used Salesforce would accumulate a higher score, while an educational nonprofit in Argentina would get a lower one.
This early score also incorporated self-reported data provided by people who signed up for a free Clearbit account. As part of the onboarding process, leads were asked how they planned to use Clearbit and could choose answers such as "find new sales prospects" and "see which companies are visiting your website.” Each response was factored into the lead score, and the most promising free signups were floated up to sales.
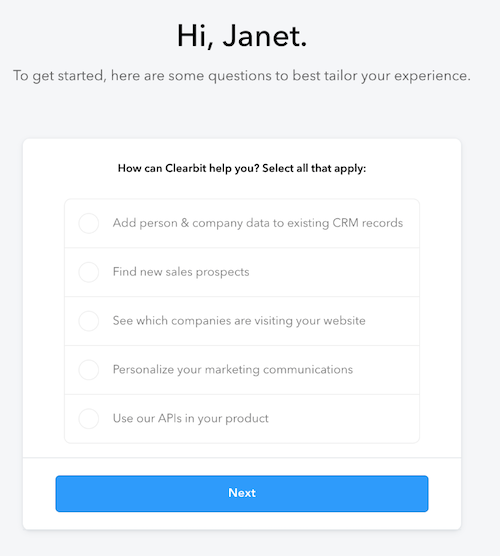
As we matured further, we stepped into a more advanced system: a predictive lead score. Today, we use MadKudu’s predictive models to score leads on fit attributes, assigning them a low, medium, good, or very good score. And for intent scoring, we use a Clearbit custom-built solution — a model that collects a lead’s behaviors as they interact with our web properties, free tools, and marketing channels.
Our scoring relies on a multitude of data points spanning firmographic, demographic, and behavioral. It’s a process that’s light years away from the early days of wondering, “Is it spam?”
3. Tools
As Clearbit’s lead scoring became more complex, our tool stack adapted to support it.
In the early days, we simply used Salesforce and Salesforce Process Builder to score and route leads. This one platform was our foundation. Now, we have a powerful network of specialized tools that we’ve integrated to support our lead qualification. For example, we use LeanData specifically for lead routing, because it’s become a complex undertaking given all our different lead types and sales team types (AE/SDR/BDR, inbound/outbound, etc.).
As a mature business, we require more data, more rules, more context, and more nuance to pull it all off and create a great, personalized experience for our diverse customer segments. Our tech stack makes room for all of that.
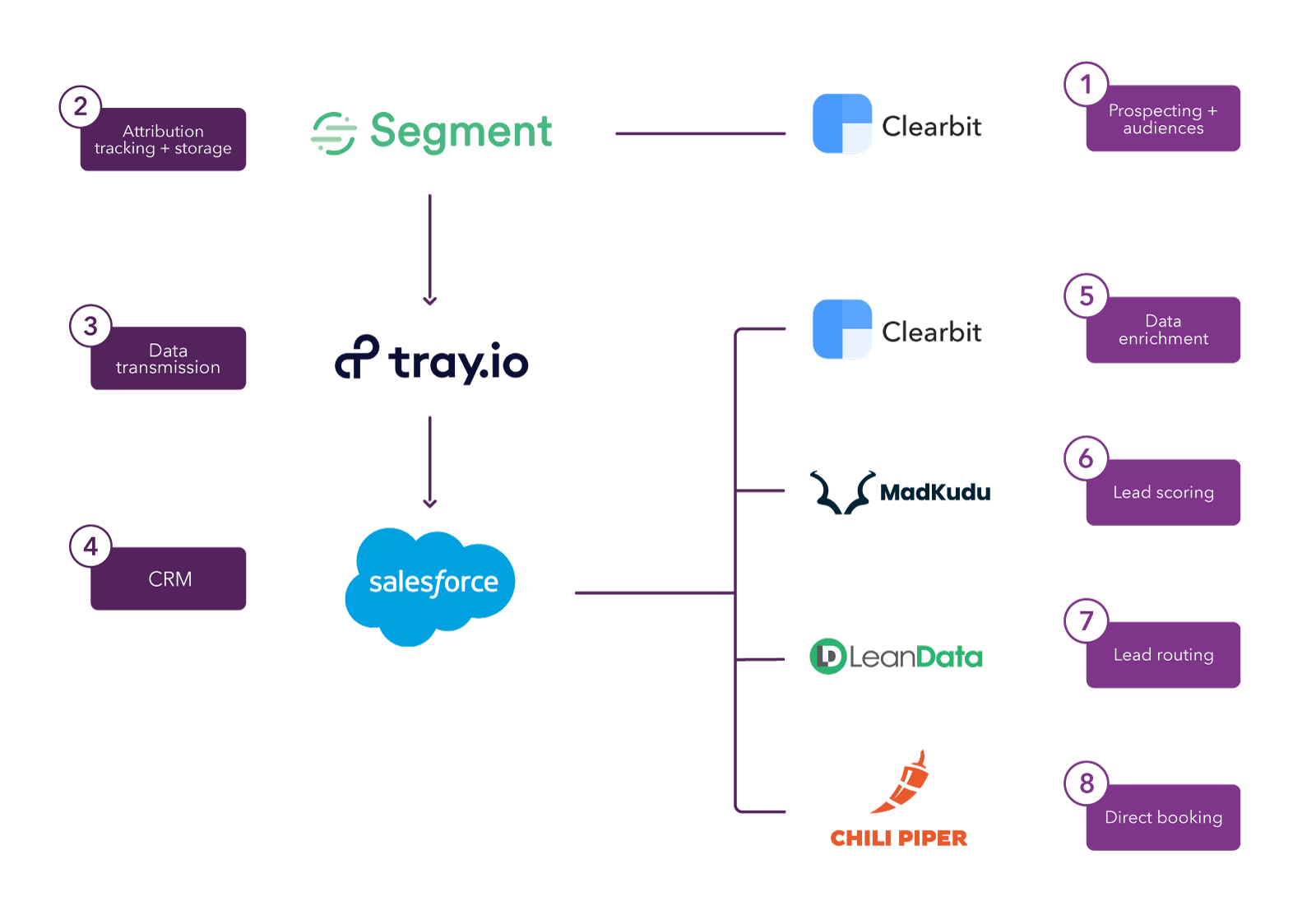
This graphic illustrates the tools that form the RevOps stack, which helps us identify new leads, score them, route them, and fast-track the best ones to a direct booking with an AE.
We also have a tech stack owned by our marketing team, which captures and makes sense of behavioral data across all leads and customers, stores the data in Redshift, and makes it available in Salesforce for our sales and CS teams to use, as well as in Customer.io so that our marketing team can send personalized emails.
Compared to using Salesforce alone, our system has maximum customizability, but it’s also more expensive to maintain — a tradeoff we chose to make.
4. ICP: Ideal Customer Profile
Our ICP also shifted over time as we introduced new products and went upmarket. One of the most interesting of these changes happened in 2020. Prior to then, our ICP was designed to predict which leads would close and make a sale. But that spring, we shifted our ICP so that it would optimize not just for leads that would close, but also for leads that would renew, expand, and provide repeat revenue.
In other words, we’ve figured out who our best customers are — they’re the ones who get the most value from Clearbit, who’ve stuck around for the long term, and have a high lifetime value. We call this type of customer our LTV ICP.
When we originally calculated which data attributes would go into our first ICP at Clearbit, we did the analysis on a list of all our closed won customers. But Clearbit wasn’t offering subscriptions at the time, and so it wasn’t until about 2019 that we had renewal data to look at. Once that data was factored in, it became clear to us that certain cohorts had a much higher lifetime value than others.
In fact, there turned out to be one predictor of high lifetime value: a company’s web traffic (the Clearbit attribute we used as a proxy was their Alexa Rank). The higher a company’s web traffic is, the more leads they have, the more noise to deal with — and the more value they find in Clearbit, because Clearbit helps them make sense of it all and know where to focus.
We leveraged this “aha” moment to shift our definition of a high-value lead and focus our energies even more effectively, knowing that certain customer segments provide a big return on investment over the long term.
Discoveries like this have influenced our ICP and helped us understand Clearbit’s market, but it’s important to note that the passage of time (in the example above, a few years’ worth of subscription data under our belt) made it possible for our lead qualification to evolve in this way.
From our ICP and lead score to our tech stack and team, we’ve gone through a lot of changes, and we don’t expect to stop. That kind of progress just comes with scaling a company and, as we like to say, we’re constantly immersed in the process of figuring it all out. What’s important is to have the resources — the tools and teams in place — to support change and to keep us agile as we grow into markets both new and old.
For a deeper discussion on how lead qualification evolves over the lifetime of a company, check out Clearbit’s Modern Guide to Lead Qualification. It’s an in-depth look at how to adjust scoring and routing to match your stages of growth, with real-life examples from SaaS companies and advice from our favorite sales, ops, and marketing practitioners.