Unlocking the Power of Your Data: How to Measure and Improve Data Quality in 6 Simple Metrics
Imagine you answer a sales call and the salesperson calls you by the wrong name. Would that sour your view of the company? Would that make you less likely to buy?
While this interaction may seem insignificant, data mistakes like this can snowball into a momentous problem for sales and marketing departments—a problem that costs businesses $13 million per year.
Knowing how to measure data quality is crucial for an organization to ensure its prospect data and other analytics are up-to-date and accurate. After all, poor data is often worse than no data at all.
For us data quality is our top priority and as data experts we want to pass along the good practices. We have a dedicated team working on that and use Telmai to optimize the monitoring and observability of the data we offer.
In this article, we will detail what data quality is, five ways to measure it, and how Clearbit’s data enrichment capabilities can aid in your organizational success.
What is data quality?
Data quality refers to the state of information an organization uses. Based on factors such as accuracy, coverage, completeness, and more, this metric helps determine whether a certain data set is fit for its intended use, or if it should be revised or updated.
This term is inherently all-encompassing and can take on a wide range of different meanings for a business. For sales and marketing departments, data quality can affect the accuracy of prospect information, advertising metrics, personalized messaging, intent-based marketing, and more.
Overall, data quality can be used to evaluate any type of data in any industry or organization.
Why is data quality important?
Data quality is important because inaccurate data can have a massive impact on a business.
Poor data quality can result in harmful events such as lost or incorrect product shipments, government fines due to incorrect financial records, and missed sales opportunities on account of inaccurate prospect information.
Speaking from a sales and marketing perspective, poor data quality not only causes missed sales opportunities, but can also limit the productivity of a sales team, cause poor customer service, and reduce the performance of personalized outreach.
To combat the effects of poor data quality, you need to ensure your data is always accurate—especially the data from third-party vendors.
Here’s a look at how you can evaluate the data quality of data intelligence providers, like Clearbit.
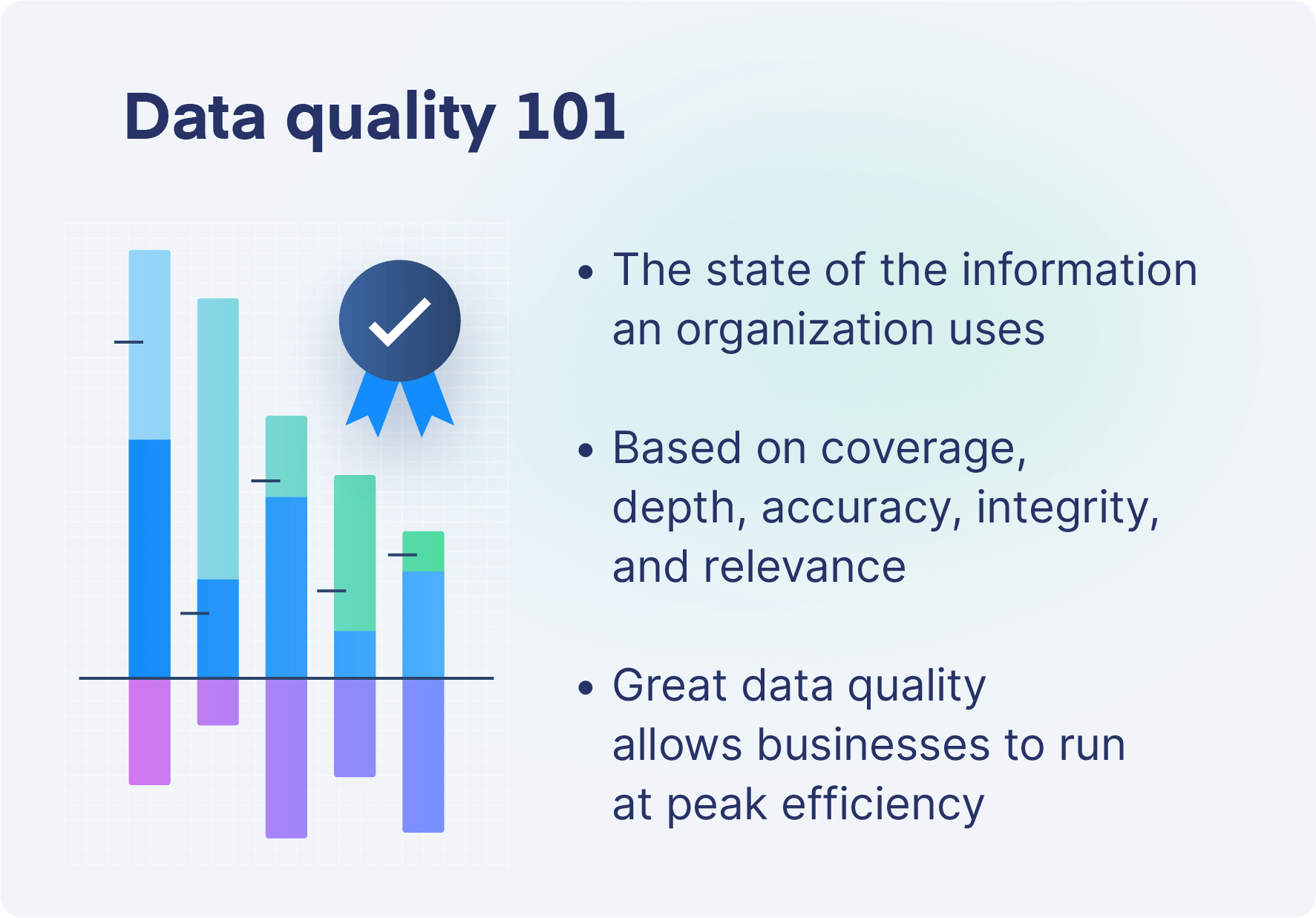
6 ways to measure data quality
Now, we will cover a few ways you can measure data quality. For relevance, we will be referring to the following data quality topics through the lens of prospect and customer data.
Before starting, the first step is to check your input data (data in your database or CRM), so you don't get bad results. The output will not be good if there are lots of invalid entries. Getting your data cleaned properly is important. For example, parsing emails to get company domains is not always accurate, as several companies use a separate email server from their website domain.
1. Coverage
Evaluating coverage is typically the easiest way to measure data quality, and it centers around the all-important match rate.
Match rate refers to the percentage of prospects that can be found—and ultimately, the amount of prospect data that can be gathered—from a data enrichment service. To evaluate the quality of coverage, simply tally up the percentage of records you submitted that were returned with data. There are, however, two variables to keep in mind.
First, evaluate how your source of information is verifying prospect data. Some platforms will fill prospect data according to a likelihood score, which may or may not be completely accurate. This scoring method tends to fill out data with calculated guesses rather than accurate information.
Second, evaluate the geographical, size and industry coverage of the prospect data. Some vendors may have excellent coverage in the United States but weak coverage globally, while others may be better at enterprise than mid-market. Base your evaluation on vendors that have a speciality in a location or company size you regularly do business with.
Finally, take a look at the invalid responses. Those will help with data cleaning. Without likelihood score, all invalid entries are discarded, and that shouldn't be confused with lower coverage.
2. Completeness
While coverage refers to the overall match rate, completeness refers to the match rate of each individual data point. These data points can include prospect name, company name, industry, and more.
The first step is to set a hierarchy for what specific prospect data points are most important for your organization. You may find, for example, that employee count is an important data point for you because you only do business with a certain company size—or, perhaps job title is a priority because you only work with executive-level employees and above.
When evaluating how complete is the data, assign a weight to each of these fields and multiply that by the corresponding match rates. By summing the resulting scores, you have an absolute rating to compare vendors with—and you can do so easily with our free data test template.
3. Timeliness
Also called Freshness, refers to how often is your data being updated. Today’s business climate is a never-ending shuffle as employees are continuously switching job titles and companies. Make sure your data is refreshing every thirty days.
4. Accuracy
Accuracy is arguably the most difficult variable to properly assess, and one that many people overlook. Inaccurate data is often worse than no data at all, so you need to take special care to ensure accuracy in your data set.
It can be painstaking, but manually going through a few hundred rows of data will ensure that your vendor is providing you with accurate information. A few special areas to be aware of include:
- Standardization: Different data points may be abbreviated, like vice president being labeled as “VP.” For this reason, you’ll probably want to review the data manually rather than comparing it exclusively with an Excel formula or similar method.
- Consistency: When evaluating the accuracy, keep an eye out for consistency in the data set. Make sure each entry is following the same informational format, be it certain abbreviations or other data presentation forms.
- Validity: when looking at the data be sure the format is the expected ones. You don’t want a letter within a numeric column breaking your logic and calculations.
- Uniqueness: take a look at duplicated records. Data without proper deduplication could looks more but is actually creating noise in your systems. You want to know for example that clearbit.co and clearbit.com is the same company.
5. Integrity
You want to make sure your data is coming from a vendor that follows all applicable business procedures in gathering that data.
Poor integrity is problematic in data quality, and can manifest in a few different ways: Either the information is willfully inaccurate in some way, or questionable ethics are used when gathering and distributing the information.
Ensure your data vendor is ethical. At Clearbit, we abide by a commitment of trust—we pledge that your data always remains your data, and we safeguard it by investing in security and honoring privacy principles as core tenets of our business.
No matter what data vendor you choose, you should confirm that they have a similar commitment to data integrity.
6. Relevance
Finally, you should be measuring your data quality on how relevant it is for your specific use case.
You could have the highest-quality data available, but if it doesn’t line up with your goals, you can likely find a better data source elsewhere. For example, your data source could have the most comprehensive information on e-commerce brands, but if your business primarily deals with other industries, that data won’t be relevant to you.
Confirm that your data is relevant for the personnel using it—and don’t be afraid to get internal opinions from your sales and marketing staff.
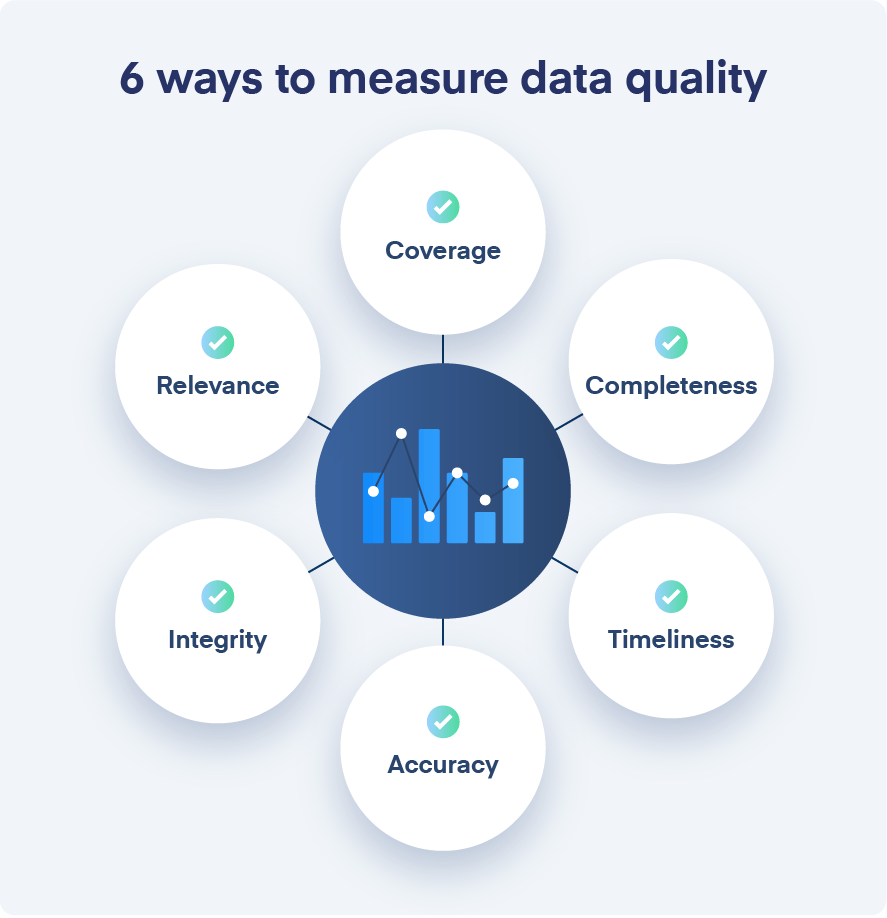
Data quality FAQ
We’ll wrap up our article with a few frequently asked questions on data quality.
What are some examples of quality metrics to track?
Two examples of quality data metrics you could track include coverage, which is the amount of prospect data that can be uncovered, and accuracy, which is how correct, timely, and consistent the data is.
What are the KPIs of quality data?
There are a few different KPIs of quality data, including:
- Coverage
- Completeness
- Timeliness
- Accuracy
- Integrity
- Relevance
Data to power your entire stack
Knowing how to measure data quality is a crucial component that keeps your organization running smoothly. Being aware of what to look for, as well as selecting the proper vendor, can make a big difference in your efficiency and ROI.
Choosing the data partner that can easily integrate into your tech stack and business process from day one should be the ultimate goal. Doing so ensures that your sales and marketing efforts aren’t sabotaged by poor data in the future.